Moore's law states that computer processing power will double every 18 months or so. There has been all sorts of extrapolations as to what this may mean to us as a society, the Singularity being one. I've got another: Gay Rights.
I did a paper back in college (late 90s) on gay marriage - I still remember the feeling of astonishment that, by that time, no state had yet allowed same-sex couples to marry. If I recall correctly, only a few allowed civil unions. As of this writing, 9 states now allow same-sex marriages, and several others are well on their way. That is a major cultural pivot in just 15 years.
My take is that the speed of the pivot has a lot to do with Moore's Law, or rather, the infrastructure it has enabled. As computer processing has grown exponentially, so too has the speed of communication. We have moved from The Pony Express to the Daily Paper to the 24-hour News Cycle to now near instant delivery, with each leap coming faster than the last. In a similar vein, social networking has expedited the sharing of opinions and thoughts among friends. What used to happen periodically on the front porch is now a constant stream. Communication is exponentially faster, and so too are its persuasive properties.
As opinions change, the impact of that change radiates with rapid speed. As one friend openly seeks to understand marriage equality, all connected friends are exposed to this shift. Even as a lone NBA player comes out as being gay, the rapid dissemination (and exploration) of this story takes over like never before. As with Moore's Law, change is happening exponentially faster.
This blog is becoming an iteration on what I am learning about. Today, it is about:
Tuesday, April 30, 2013
Friday, April 26, 2013
David Brooks, Your Premise is Off!
I've already blogged about some of David Brooks' writing on big data. Though it is admirable that he is taking the time to delve in to the emerging world of data, he needs to apply some differential thinking to the information he is collecting. In this piece, his premise is again off:
The power of data is in the information it contains, not the method by which it is extracted. And the limit is our imagination.
The theory of big data is to have no theory, at least about human nature. You just gather huge amounts of information, observe the patterns and estimate probabilities about how people will act in the future.This is not the theory of big data - this is a small sliver of what is and can be done with the explosion of structured data that is popping around us. To diminish the power of big data to just what can be gleaned through "estimated probabilities" is to focus on the tree and not the forest.
The power of data is in the information it contains, not the method by which it is extracted. And the limit is our imagination.
Thursday, April 25, 2013
The Philosophy of Data
In this article titled, "The Philosophy of Data", auther David Brooks asks:
I think this is the wrong question to ask. This may be a bit optimistic, but my belief is that data analyses can answer most any question. The problem (and opportunity) lies in ensuring the data contains the necessary information to answer the question - a problem we have only begun to explore.
In the same article:
What kinds of events are predictable using statistical analysis and what sorts of events are not?Now, I know an editor likely created the title, but his article limits the value of data to insights derived from statistical analysis - as if that is the only means to extract information from (big) data.
I think this is the wrong question to ask. This may be a bit optimistic, but my belief is that data analyses can answer most any question. The problem (and opportunity) lies in ensuring the data contains the necessary information to answer the question - a problem we have only begun to explore.
In the same article:
...we tend to get carried away in our desire to reduce everything to the quantifiable.Data is not just about quantification; it's about information. We are only at the beginning of collecting, structuring, and even analyzing data. My belief is that we will see great advances in this processing, which will in turn unlock new possibilities for data-driven insights. Such innovation will enable analyses and insights never before possible. Data will inform questions we don't even yet know to ask.
Wednesday, April 24, 2013
Big Data and Hiring
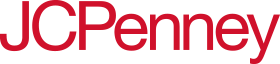
I spent some time at PeopleAnswers in it's early days (I was employ #3!) - a business that has scaled behavioral testing to improve hiring and recruitment. They have (very effectively) attacked part of the problem - exposing our innate selves that drive behavior to potential hiring managers. This innateness is the foundation of our potential succes. But it is not our whole selves - our experience, our passions, and other variables also play a role in determining our career success.
I wonder what a systemic understanding of JC Penney, Target and Apple's characteristics, culture, products, etc., might have told the JC Penney board, when coupled with Ron Johnson's behavioral profile and experience? Might they have seen the mismatch sooner?
Friday, April 19, 2013
Big Data and a Portfolio Approach
Given the sharp decline of the cost of data storage and the emergence of scalable tools to explore and mine this data, more and more data is becoming systemically accessible every day. We are just at the beginning of applying the information available among the growing data sets around us.
Big data is, well, big. It is new. And the tools emerging to access and harvest the information it contains are also new. Therefore, getting to real, useful information when exploring big data is a difficult task.
Many of the applications of big data have to been big as well. And complex. This complexity of application on top of what is already a complex myriad of nascent tools makes for a very brittle system.
I've been thinking about this differently. My take is that we need to focus engineering lift on the complex methods and tools to extract information from data, and streamline and simplify the application. Simple applications are easier and faster to build. Faster builds allow for a quicker return on effort. Product designers should therefore focus on thin web apps that leverage these vast, complex datasets. Think of your initial applications as prototypes for your big data system...
How can we use big data in small, focused ways to improve our lives? What "little" things can be extracted from available datasets and applied quickly? How can the burdens of complexity be pushed down the stack, to simplify the application, and lessen the investment required before reaping any value?
Oh, and one more thing - there is another benefit to pushing as much of the engineering and complexity to the data processing layer. This also enables a portfolio approach, whereby tens, hundreds or even thousands of apps can be built on a single data stack. Why use a shot gun or even a sniper, when you can use an army to mine for value...
UPDATE: I just started playing with a new app that munges this thinking, Osito. (Good overview from The Verge here.) Basically, it's a single iOS app that leverages the portfolio approach to provide lighter, thin alerts given your personal data. The product focus is triggers based on user location. Interesting play - we'll see if it works...
Big data is, well, big. It is new. And the tools emerging to access and harvest the information it contains are also new. Therefore, getting to real, useful information when exploring big data is a difficult task.
Many of the applications of big data have to been big as well. And complex. This complexity of application on top of what is already a complex myriad of nascent tools makes for a very brittle system.
I've been thinking about this differently. My take is that we need to focus engineering lift on the complex methods and tools to extract information from data, and streamline and simplify the application. Simple applications are easier and faster to build. Faster builds allow for a quicker return on effort. Product designers should therefore focus on thin web apps that leverage these vast, complex datasets. Think of your initial applications as prototypes for your big data system...
How can we use big data in small, focused ways to improve our lives? What "little" things can be extracted from available datasets and applied quickly? How can the burdens of complexity be pushed down the stack, to simplify the application, and lessen the investment required before reaping any value?

UPDATE: I just started playing with a new app that munges this thinking, Osito. (Good overview from The Verge here.) Basically, it's a single iOS app that leverages the portfolio approach to provide lighter, thin alerts given your personal data. The product focus is triggers based on user location. Interesting play - we'll see if it works...
Thursday, April 18, 2013
Data Science vs. Data Intelligence
Sean Gourley gave a very interesting talk at GigaOm's Structure Data conference last month. I have repeated his ideas around data science vs. data intelligence in several conversations. (Stacey Higginbotham does a great job distilling the talk here - the full talk is embedded below.)
He lays out the idea in one simple chart:
He also provides a few rules of the road about data:
He lays out the idea in one simple chart:
He also provides a few rules of the road about data:
- Data needs to be designed for human interaction
- Understand limits of human processing
- Data is messy, incomplete, and biased
- Data needs theory
- Data needs stories... Stories need data
I have seen first hand bubble-like aspirations for what "big data" plus "data science" can offer. Because the technologies are new, and so many are now becoming aware of the power of high-end statistics and machine learning, data science is perceived to be larger than it actually is for may.
It is a tool, a method to solve problems big and small. It isn't an answer.
Wednesday, April 17, 2013
Dusting it off...
After a multi-year hiatus, I am dusting off the blog. Since my last note, my company (Nico Networks) was purchased by The Washington Post Company. My partner and I joined what became WaPo Labs. This move afforded me the opportunity to operationalize my thoughts and ideas faster than I could document them (how's that for an excuse?).
Through this experience, we were able to iterate and expand upon many of the ideas discussed to date in this blog. However, instead of being limited to political campaigning, we were afforded the resources of one of the largest media organizations in the world. As opportunity expanded, so too did the ideas...
Reflecting back, a common thread throughout has been the application of information culled from data. The earliest applications we did on behalf of our largest client, Catalist, were built upon their voter file data. At Labs, the team continues to expand and iterate on the application of information extracted from past activity streams, and from deep text analytics happening on the company's vast corpus of content built over decades. We accomplished a great deal, and I am excited to see what continues to come from what is an amazing and very talented group of innovators.
We are only at the beginning of what has already been coined as the information economy. The technology and expertise to efficiently extract usable information from the growing data sets that are emerging around the world is just being discovered. As more data becomes structured, more interesting and never-before-seen deductions and associations can be made. As new technologies and capabilities are applied, new stories can be told.
The brave new world is emerging, and I am excited to be a part of it, to continue to explore how information from data can change the world. More to come...
Through this experience, we were able to iterate and expand upon many of the ideas discussed to date in this blog. However, instead of being limited to political campaigning, we were afforded the resources of one of the largest media organizations in the world. As opportunity expanded, so too did the ideas...
Reflecting back, a common thread throughout has been the application of information culled from data. The earliest applications we did on behalf of our largest client, Catalist, were built upon their voter file data. At Labs, the team continues to expand and iterate on the application of information extracted from past activity streams, and from deep text analytics happening on the company's vast corpus of content built over decades. We accomplished a great deal, and I am excited to see what continues to come from what is an amazing and very talented group of innovators.
We are only at the beginning of what has already been coined as the information economy. The technology and expertise to efficiently extract usable information from the growing data sets that are emerging around the world is just being discovered. As more data becomes structured, more interesting and never-before-seen deductions and associations can be made. As new technologies and capabilities are applied, new stories can be told.
The brave new world is emerging, and I am excited to be a part of it, to continue to explore how information from data can change the world. More to come...
Subscribe to:
Posts (Atom)